Habitat-Web: Learning Embodied Object-Search Strategies from Human Demonstrations at Scale
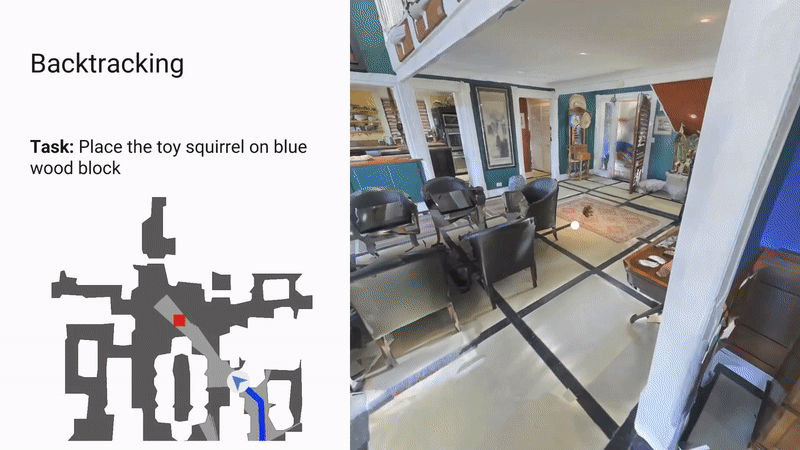
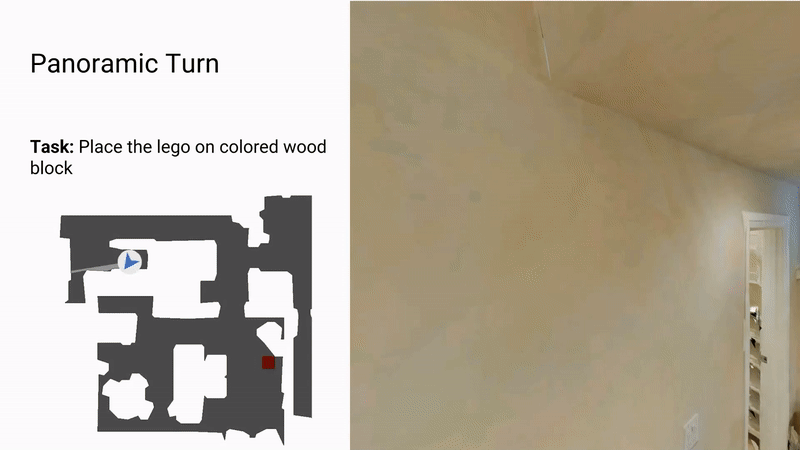
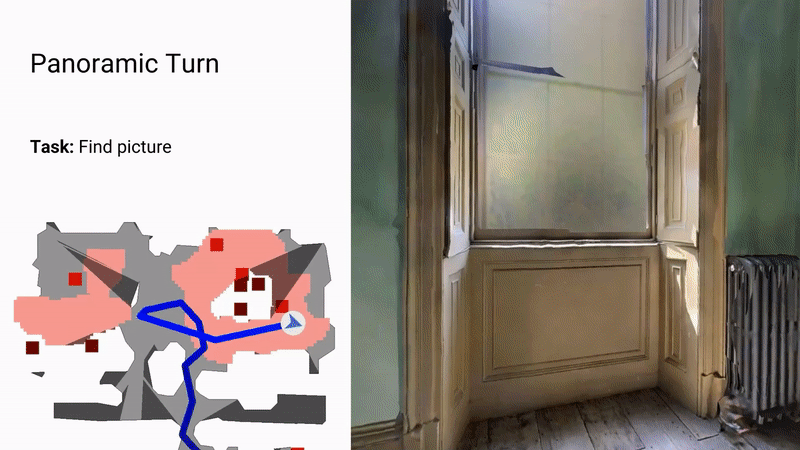
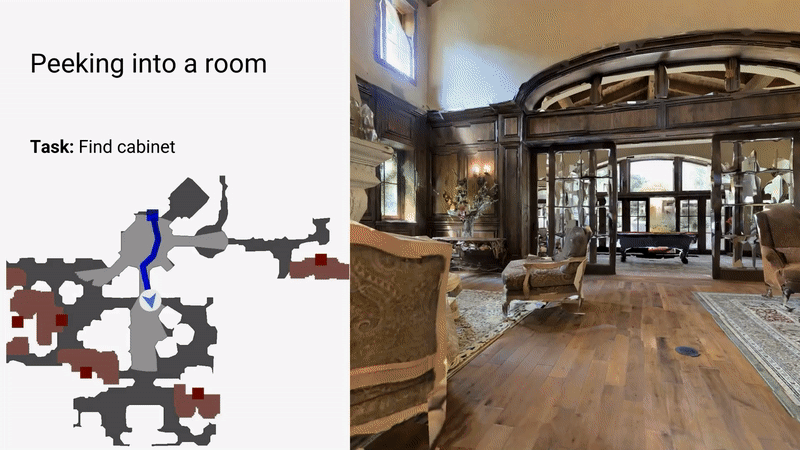
Visualization of learnt agent behaviors
In this paper, we present a large-scale study of imitating human demonstrations on tasks that require a virtual robot to search for objects in new environments -- (1) ObjectGoal Navigation (e.g. find & go to a chair) and (2) PickPlace (e.g. find mug, pick mug, find counter, place mug on counter). Towards this we collect a large scale dataset of 70k human demonstrations for ObjectNav and 12k human demonstrations for PickPlace tasks using our web infrastructure Habitat-Web. We use this data to answer the question - how does large-scale imitation learning (IL) compare to large-scale reinforcement learning (RL)? On ObjectNav we find that IL using only 70k human demonstrations outperforms RL using 240k agent gathered trajecotries by 3.3% on success and 1.1% on SPL. On PickPlace, the comparison is even starker - IL agent achieves ~18% success on episodes with new object-receptacle locations while RL agent fails to get beyond 0% success. More importantly, we find that IL-trained agents learn efficient object-search behavior from humans - it peeks into rooms, checks corners for small objects, etc.
Read more in the paper.
Short Presentation
Paper
@inproceedings{rramrakhya2022, title={Habitat-Web: Learning Embodied Object-Search Strategies from Human Demonstrations at Scale}, author={Ram Ramrakhya and Eric Undersander and Dhruv Batra and Abhishek Das}, year={2022}, booktitle={CVPR}, }
Code and Data
Acknowledgements
We thank Devi Parikh for help with idea conceptualization. The Georgia Tech effort was supported in part by NSF, ONR YIP, and ARO PECASE. The views and conclusions contained herein are those of the authors and should not be interpreted as necessarily representing the official policies or endorsements, either expressed or implied, of the U.S. Government, or any sponsor.